How AI and Producer Performance Data Can Help Insurers Improve Renewal Rates
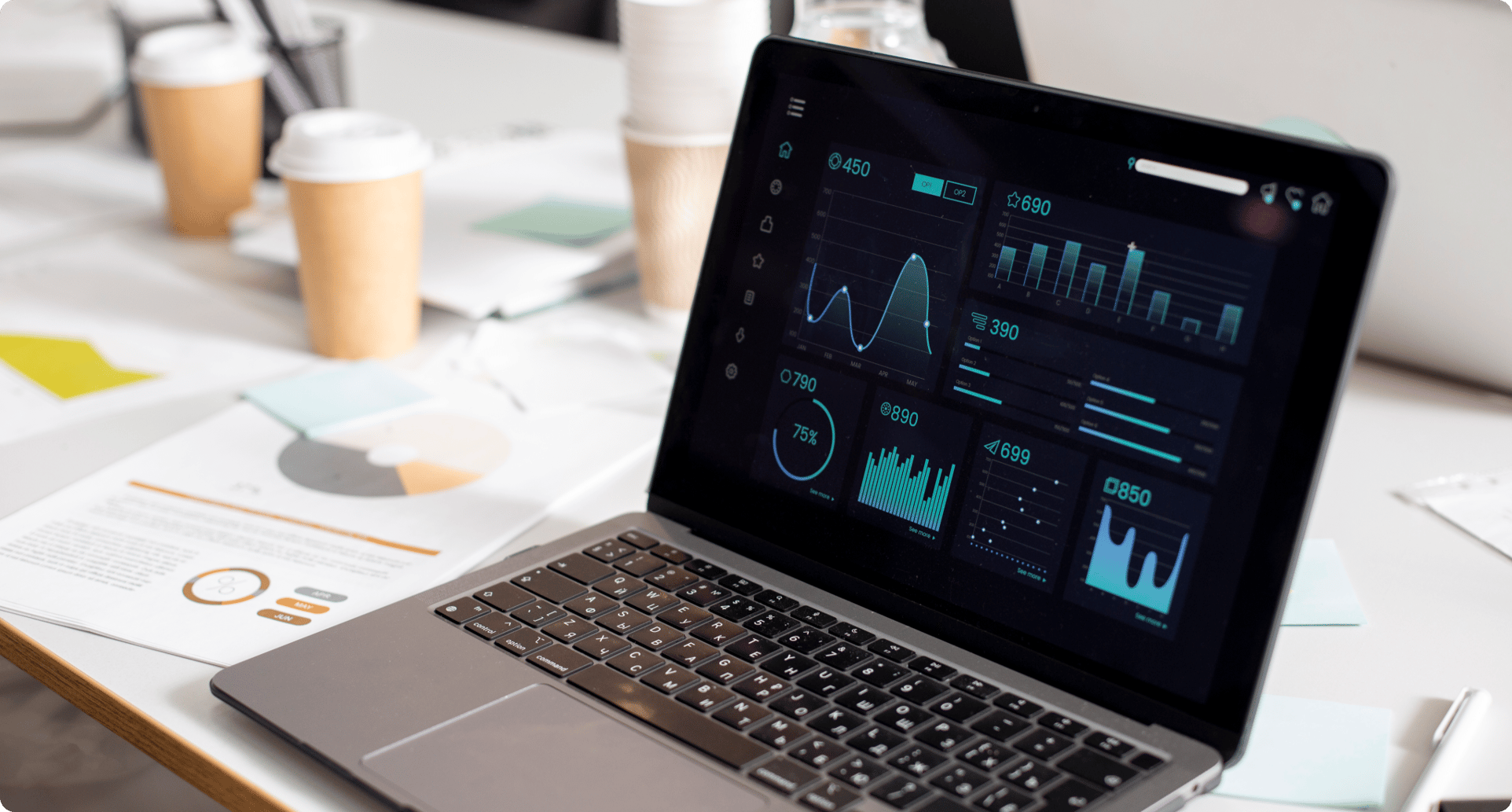
In today's uber competitive insurance landscape, every advantage matters. Insurers are constantly seeking opportunities to gain a competitive edge, and reducing policyholder churn is a prime focus area. Let's explore how leveraging producer performance data can provide insurers with a significant advantage in this regard.
Understanding Customer Behavior:
Producer record data encompasses a wealth of information regarding customer interactions, preferences, and historical policy data. This includes details such as past purchases, policy usage, and communication history.
AI algorithms can process this data to uncover intricate patterns and trends in customer behavior. By examining factors like engagement levels, claims history, and policy renewal trends, insurers gain a nuanced understanding of each customer's preferences and likelihood to renew.
Personalized Renewal Offers and Recommendations:
Producer record data provides insights into customers' current policies, coverage levels, and renewal dates. This information allows insurers to craft tailored renewal offers that align with each customer's unique needs and preferences.
Through AI-powered segmentation and analysis, insurers can categorize customers based on various criteria such as risk profiles, demographics, and historical behavior. This enables the delivery of personalized renewal offers and recommendations that resonate with each segment, thereby increasing the likelihood of renewal.
Proactive Engagement Strategies to Mitigate Churn:
By tracking customer interactions and engagement levels with producers, insurers can identify early signs of potential churn or non-renewal. This includes monitoring changes in communication patterns, policy usage, and customer inquiries.
AI algorithms can predict churn probabilities by analyzing patterns in customer behavior and historical renewal outcomes. Armed with this insight, insurers can implement proactive engagement strategies, such as targeted outreach campaigns or personalized retention offers, to mitigate churn and encourage policy renewal.
Predictive Modeling for Renewal Probability Assessment:
By leveraging historical policy data and customer information from producer records, insurers can build predictive models to assess the likelihood of policy renewal for each customer.
AI-driven predictive modeling algorithms analyze diverse data points, including customer demographics, claims history, and satisfaction metrics. By forecasting renewal probabilities with precision, insurers can prioritize renewal efforts and resources towards customers with the highest likelihood of renewing their policies.
Continuous Improvement Through a Data-Driven Feedback Loop:
Producer record data captures feedback from customers, producers, and internal stakeholders regarding the renewal process and overall customer experience. This includes insights into pain points, satisfaction levels, and areas for improvement.
By using AI-powered sentiment analysis, insurers can sift through vast amounts of feedback data to uncover actionable insights. By identifying common themes, trends, and specific pain points, insurers can pinpoint areas for enhancement within the renewal process.
Armed with these insights, insurers can implement iterative improvements to the renewal process, including streamlining workflows, enhancing communication channels, and optimizing renewal offers. This continuous feedback loop ensures that renewal strategies evolve in alignment with changing customer needs and market dynamics, ultimately driving higher renewal rates and customer satisfaction levels over time.
In summary, leveraging producer record data and AI-driven insights allows insurers to create personalized renewal strategies, proactively engage with customers, and continuously refine their practice based on feedback. This holistic approach not only enhances renewal rates, but also fosters long-term customer loyalty and profitability in the competitive insurance landscape.